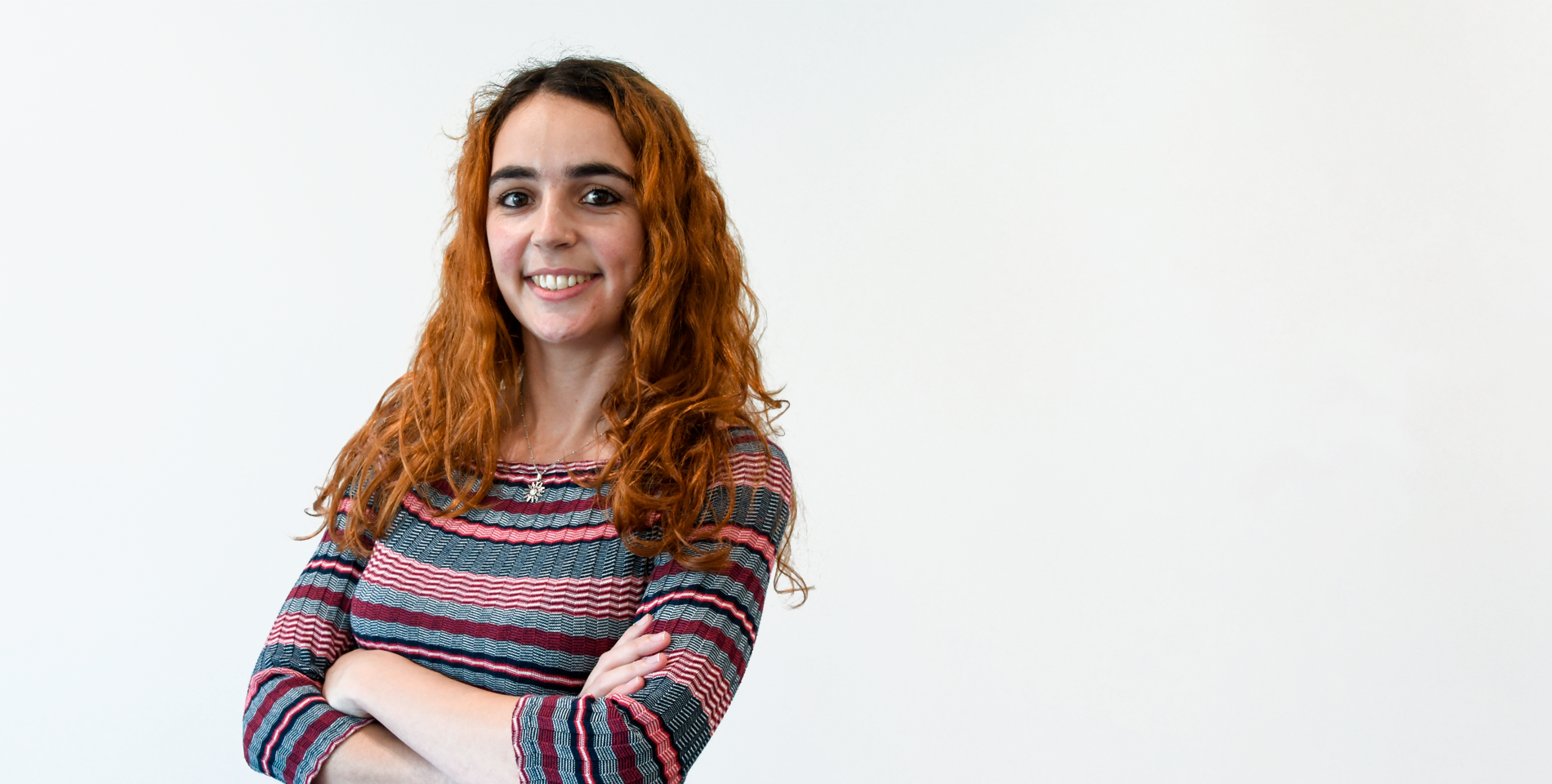
Enhancing autonomous detection algorithms in NDT strategies for the future: An interview with Joana Ramos
In this interview, we will delve into the strategies aimed at enhancing autonomous detection algorithms and explore how we can progress toward an even more precise and efficient future in this constantly evolving field. To accompany us on this exciting journey of discovery, we will have the valuable insights and expertise of Joana Ramos, Project Manager and NDT specialist at Endity.
/ Let’s start by exploring the advantages of autonomous detection algorithms compared to traditional approaches. What are the main advantages you have found in your experience?
Sure, the advantages of autonomous detection algorithms are notable. Firstly, these algorithms are particularly useful when it comes to dealing with large volumes of data, as artificial intelligence is inherently efficient in handling large datasets.
Secondly, the evaluation of these algorithms is considerably faster than traditional methods, which significantly reduces the time needed for inspection. This is crucial for production and maintenance efficiency.
Finally, autonomous detection algorithms are dynamic models, which means that they can adapt and retrain themselves in case manufacturing and maintenance conditions change. This adaptability is an important advantage in constantly evolving environments.
/ Very interesting. Now, let’s talk about the limitations or drawbacks of these algorithms. What are some of the challenges you face compared to traditional approaches?
Of course, there are no perfect solutions. Autonomous detection algorithms have some disadvantages that need to be addressed. Firstly, they require a learning and training phase, and this can sometimes be complicated if not enough training parts or data are available at the beginning of the process.
The implementation phase can also be challenging, as the model needs to be adjusted to the reality of production and maintenance. This may require additional time and resources to ensure adequate adaptation.
/ Let’s start by explaining how the training of an autonomous detection algorithm is carried out. What are the key steps in this process?
The training process of an autonomous detection algorithm involves several critical steps. We use the CRISP-DM (Cross Industry Standard Process for Data Mining) method. This methodology proposes four essential steps that must be completed between problem conceptualisation and final implementation: data acquisition, data pre-processing, model training and model evaluation.
The acquisition phase involves collecting the necessary inspection data according to the defined inspection system. This data acts as the basis for training.
The pre-processing phase, which is fundamental. Here, the data is labelled according to the good part/bad part criterion. The data set is also divided into the appropriate partitions: training, validation and testing. This is essential to evaluate the performance of the model effectively.
The training phase is where machine learning is applied. The artificial intelligence algorithm creates a prediction model that relates the input features to the target variable. The model architecture and hyperparameters are adjusted based on the results obtained during training to achieve the best possible prediction.
Finally, in the evaluation phase, metrics are used to select the model that best solves the problem at hand.
/ Excellent explanation, is there any advice you can give to those who are looking to implement such algorithms in their operations?
My advice would be to start with a clear understanding of your problem and keep in mind that training and improving autonomous detection algorithms is an iterative process.
The importance of the data pre-processing phase should not be underestimated, as the quality of the data has a significant impact on the performance of the model.
/ What are the hardware and software requirements needed to effectively implement autonomous sensing algorithms?
Effective implementation of autonomous detection algorithms is essential in environments such as Endity’s, where an automated NDT solution based on AI models is sought.
In terms of hardware and software, the systems Endity works with for eddy current (EC) and ultrasonic (UT) inspection are ready to transform inspection data into useful information for AI models. These systems typically have the necessary processing power and storage capabilities to work with machine learning algorithms.
In terms of software, it is essential to have a processor powerful enough to handle large volumes of data and run AI models efficiently. In addition, specialised software will be required that can load, run and evaluate these models effectively.
In short, you need accurate hardware capable of detecting complex defects and processors that can efficiently handle large volumes of data and run AI models efficiently. On the software side, appropriate development and data management tools are essential to implement and operate the algorithms effectively in the context of Endity.
endity